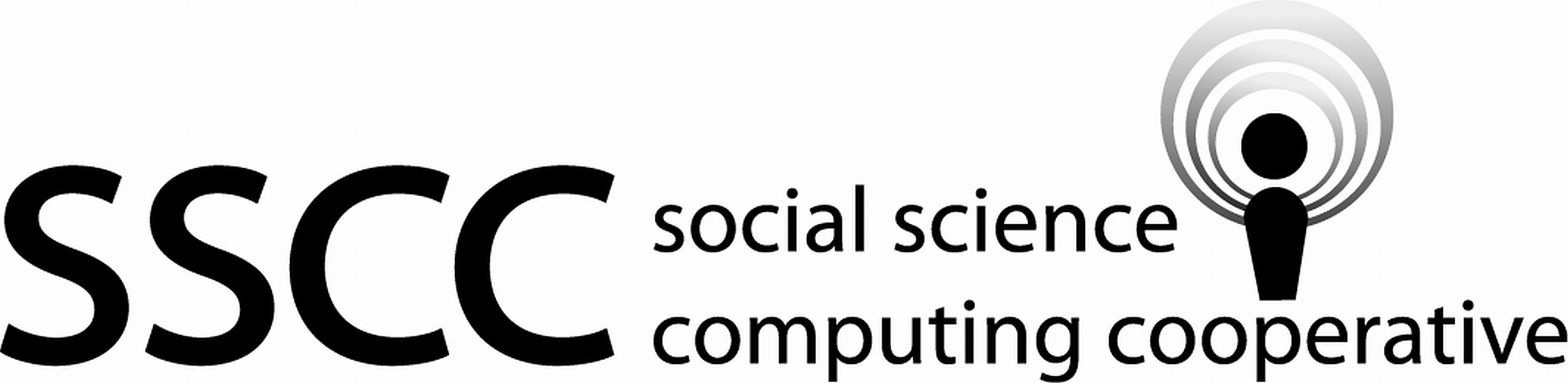
4.1 Preparatory exercises
The skills in these exercise are used in the exercises at the end of the discourses of this chapter. Take a moment and complete these to confirm that you are prepared for this chapter. If these exercises are difficult, review the prior chapters.
Import the
PSID.csv
data set.The following is used at the RStudio prompt to enter Python mode.
library(reticulate) repl_python()
The remainer is Python code.
from pathlib import Path import pandas as pd import plotnine as p9
psid_path = Path('..') / 'datasets' / 'PSID.csv' psid = pd.read_csv(psid_path) print(psid.dtypes)
Unnamed: 0 int64 intnum int64 persnum int64 age int64 educatn float64 earnings int64 hours int64 kids int64 married object dtype: object
Plot
earnings
versehours
.print( p9.ggplot(psid, p9.aes(x='hours', y='earnings')) + p9.geom_point() + p9.theme_bw())
<ggplot: (143591241869)>
Make a boxplot of
earnings
with separate boxplots for eachmarried
status.print( p9.ggplot(psid, p9.aes(x='married', y='earnings')) + p9.geom_boxplot() + p9.theme_bw())
<ggplot: (143591233586)>
Make a horizontal boxplot of
earnings
with separate boxplots for eachmarried
status.This should be the same plot as in the prior example only the earnings are displayed on the horizontal axis.
This is useful when there are many boxplots or the category names are long.
print( p9.ggplot(psid, p9.aes(x='married', y='earnings')) + p9.geom_boxplot() + p9.coord_flip() + p9.theme_bw())
<ggplot: (-9223371893263501845)>
Do all of the categories of married make sense?
The
NA/DF
andno histories
would make more sense being combined into a single set ofNA
observations.Plot
earnings
versekids
.print( p9.ggplot(psid, p9.aes(x='kids', y='earnings')) + p9.geom_point() + p9.theme_bw())
<ggplot: (143591209501)>
What can be learned from this plot?
There appears to a number of observations that have akids
value of over- These are likely a code for
NA
.
This would be more informative if earnings were displayed as a boxplot for each number of
kids
.- These are likely a code for