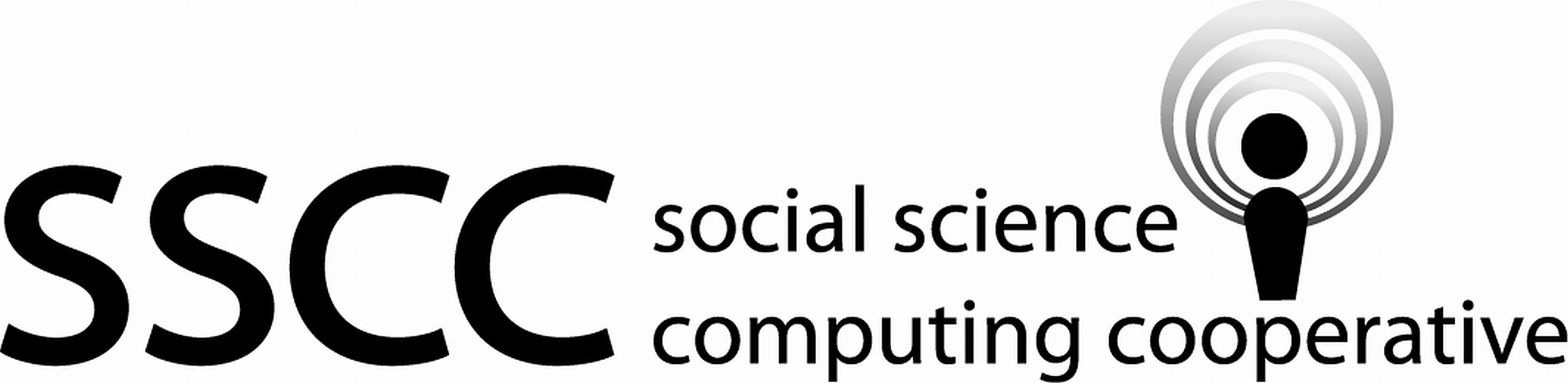
4.5 Dropping unneeded observations
These exercises use the PSID.csv
data set
that was imported in the prior section.
Import the
PSID.csv
data set.from pathlib import Path import pandas as pd
psid_path = Path('..') / 'datasets' / 'PSID.csv' psid_in = pd.read_csv(psid_path) psid_in = ( psid_in .rename( columns={ 'Unnamed: 0': 'obs_num', 'intnum': 'intvw_num', 'persnum': 'person_id', 'married': 'marital_status'})) psid = psid_in.copy(deep=True) psid = psid.drop(columns='obs_num') print(psid.dtypes)
intvw_num int64 person_id int64 age int64 educatn float64 earnings int64 hours int64 kids int64 marital_status object dtype: object
Display some of the observations where there are more than 90 kids in the household. Chose several of the pertinent variables to display.
(psid .query(' kids > 90') .loc[:, ['person_id', 'age', 'educatn', 'kids', 'marital_status']] .head(n=15) .pipe(print))
person_id age educatn kids marital_status 10 3 48 13.0 98 divorced 150 186 41 12.0 98 married 323 178 49 12.0 98 married 357 5 34 99.0 99 no histories 447 3 34 12.0 98 divorced 544 2 47 12.0 98 divorced 590 182 49 12.0 99 no histories 739 3 48 3.0 98 never married 749 21 49 0.0 99 no histories 857 177 40 0.0 98 married 1027 3 45 12.0 98 married 1076 2 50 0.0 99 no histories 1167 171 49 0.0 98 divorced 1174 173 40 9.0 98 divorced 1187 175 37 0.0 98 divorced
Create a copy of the data frame that removes the observations where
married
wasno history
orNA/DF
. You may have combined these categories into a missing category in the preparatory exercises.psid_copy = ( psid.query( 'marital_status == "no history" | marital_status == "NA/DF"')) (psid_copy .loc[:, ['person_id', 'age', 'educatn', 'kids', 'marital_status']] .head(n=15) .pipe(print))
person_id age educatn kids marital_status 1665 3 45 17.0 0 NA/DF 1843 3 38 12.0 1 NA/DF 2240 174 36 17.0 0 NA/DF 2244 177 32 14.0 1 NA/DF 2840 4 46 14.0 0 NA/DF 2971 9 31 14.0 2 NA/DF 3563 2 46 12.0 0 NA/DF 3643 4 30 11.0 2 NA/DF 3818 174 41 99.0 0 NA/DF